ANR MAKIMONO
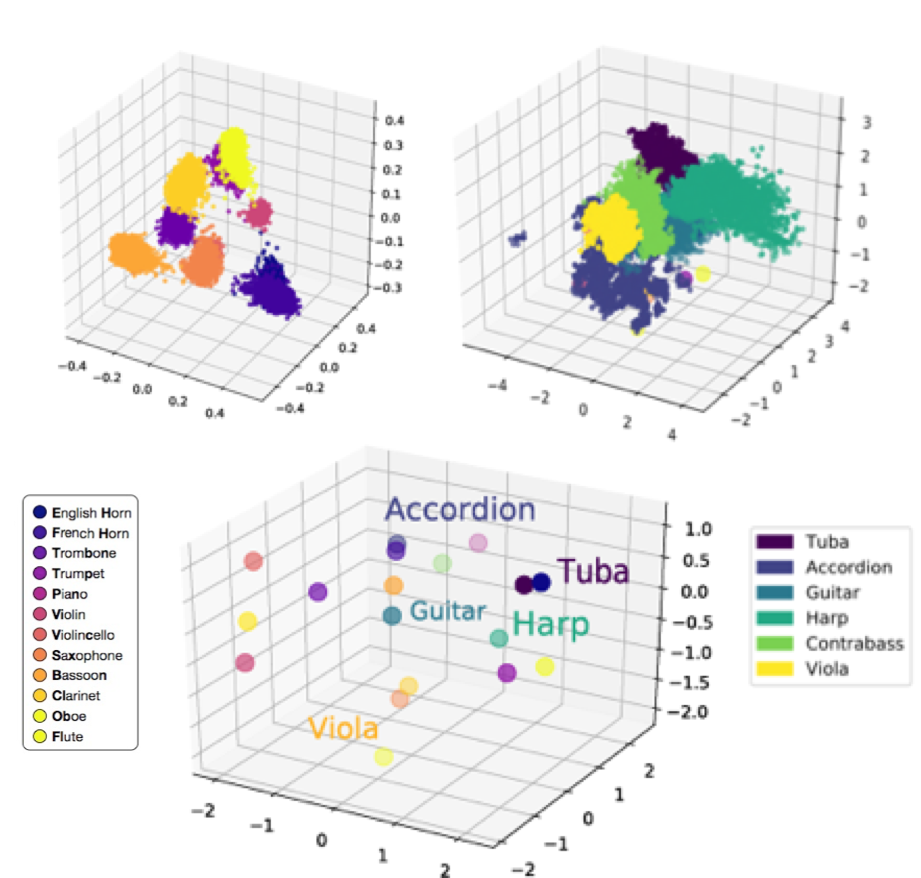
This project is a collaborative venture between IRCAM, McGill University, and the OrchPlayMusic company, co-funded by the French ANR, and the Canadian SSHRC.
INITIAL OBJECTIVES OF THE PROJECT
The initial objectives of the project are to transform musical orchestration by targeting the emerging properties of instrumental mixtures in terms of perceptual effects, the multimodal sources of information in orchestration linking the spectral (instrumental properties), symbolic (musical writing) and perceptual (timbre perception) information. To provide the computational expertise of orchestration practice and perception (through well-known but under-defined orchestral effects) by developing innovative machine learning algorithms targeted at perceptual effects, but also to extract rich pedagogical knowledge for the practice of orchestration. The main objectives within the time frame of this proposal are:
-
model human perception as a rational basis for understanding intricate phenomena, through optimal acoustic and symbolic representations of perceptual effects in orchestral music as a basis for learning;
-
devise novel learning algorithms for multimodal embedding spaces that will allow for the deciphering of joint signal-symbol interactions underlying the perceptual orchestral effects;
-
perform semantic inference and knowledge extraction in order to exhibit patterns and metric relationships between modalities and known orchestral examples, but also to generate novel music
-
to validate both the learned spaces and extracted knowledge by performing extensive perceptual experiments, but also through pedagogical and compositional applications.
By closing the loop between perceptual effects, their analyses and a perceptual validation of the extracted knowledge, this project aims to provide enhancement in the targeted fields but also generic methods broadly applicable to other scientific fields. The project will produce a set of software and models as well as experimental data shared publicly. The partnership aims to produce digital tools for solving orchestration problems and analyses of musical scores and treatises.
DESCRIPTION
Orchestration is the subtle art of writing music by combining the properties of different instruments in order to achieve various sonic goals. This complex skill has an enormous impact on classical, popular, film and television music, and video game development.
For centuries, orchestration has been (and still is) transmitted empirically through treatises of examples by focusing solely on describing how composers have scored instruments in pieces rather than understanding why they made such choices.
Hence, a true scientific theory of orchestration has not yet emerged and needs to be addressed systematically, even though the obstacles such an analysis and formalization must surmount are tremendous. This project aims to develop the first approach to the long-term goal of a scientific theory of orchestration by coalescing the domains of computer science, artificial intelligence, experimental psychology, signal processing, computational audition, and music theory.
To achieve this aim, the project will exploit a large dataset of orchestral pieces in digital form for both symbolic scores and multi-track acoustic renderings of independent instrumental tracks.
This set is continuously expanding through a leveraged research project, in which the partners of are also main collaborators.
Orchestral excerpts have been and are currently being annotated by panels of musician experts, in terms of the occurrence of given perceptual effects related to auditory grouping principles.
This leads to an unprecedented library of orchestral knowledge, readily available in both symbolic and signal formats for data mining and deep learning approaches.
We intend to harness this unique source of knowledge, by first evaluating the optimal representations for symbolic scores and audio recordings of orchestration based on their predictive capabilities for given perceptual effects. Then, we will develop novel learning and knowledge extraction methods based on the discovered representations of perceptual effects in orchestration. To this end, we will develop deep learning methods able to link audio signals, symbolic scores, and perceptual analyses by targeting multimodal embedding spaces (transforming multiple sources of information into a unified coordinate system).
These spaces can provide metric relationships between modalities that can be exploited for both automatic generation and knowledge extraction.
The results from these models will then feed back to the analysis of orchestration and will also be validated through extensive perceptual studies.
These results will in turn enhance the organization of the knowledge database, as well as the software of our collaborating industrial partner OrchPlayMusic.
By closing the loop between perceptual effects and learning, while validating the higher-level knowledge that will be extracted, this project will revolutionize creative approaches to orchestration and its pedagogy.
The predicted outputs include the development of the first technological tools for the automatic analysis of orchestral scores, for predicting the perceptual results of combining multiple musical sources, as well as the development of prototype interfaces for orchestration pedagogy, computer-aided orchestration and instrumental performance in ensembles.
RESEARCH PROGRAM
This project seeks to create novel representations and learning and analysis techniques to establish a scientific basis for musical orchestration practice.
The objectives are to provide computational analyses of orchestration practice and perception (through well-known but under-defined orchestral effects) by developing innovative machine learning algorithms in order to understand higher-level perceptual effects, but also to obtain a rich pedagogical knowledge to :
- Analyze the optimal acoustic and symbolic representations of orchestral music to serve as a basis for both further learning algorithms and advanced perceptual experiments;
- Develop machine learning algorithms (based on different types of information) for multimodal embedding spaces to decipher the signal-symbol relations;
- Perform semantic inference from these spaces in order to study the underlying relationships between modalities, but also to perform generation of novel musical content; and
- Validate both the learned spaces and extracted knowledge by performing extensive perceptual experiments and developing pedagogical and compositional applications.
Therefore, our first objective is to develop optimal symbolic and signal representations of orchestral music.
By evaluating the predictive power of these representations, we will orient further investigations on perceptual properties.
By performing a coordinated analysis between the musical scores, separate audio recordings of different instruments and perceptual annotations, we can identify the relationships between these sets of information.
Therefore, we will develop learning algorithms to find embedding spaces that link these different modalities into a unified coordinate system.
We will perform knowledge extraction, inference and generation of one modality based on the others.
Finally, the results from these models will be validated through extensive perceptual studies that will continuously feed back to the other tasks.
SIGNIFICANT EVENTS AND RESULTS
-
Creation and funding of the ACTOR partnership. Thanks to the original funding provided by this project and the original partners (McGill, IRCAM), we successfully obtained a network partnership grant from the Canadian NSERC, creating the Analysis, Creation and Teaching of Orchestration (ACTOR) partnership (https://www.actorproject.org/). This partnership includes 19 partners from 9 countries and provides up to 1.5 M$ funded over 7 years.
-
Several further funding projects awarded. In the continuity of this project work, several funding were awarded to different French partners of the project. Notably, the ANR MERCI (G. Assayag, ~400k€), the Emergence(s) Sorbonnes ACIMO (P. Esling, ~100k€) and the Emergence(s) Ville de Paris ACIDITEAM (P. Esling, ~500k€) are all based on some of the results from this project.
-
Best presentation award at ISMIR 2018. The work performed around unsupervised learning of variational generative models regularized by perception [Esling et al. 2018] would have been impossible without the data and help from our foreign partner, which provided experience and data from their previous studies [McAdams et al. 2002]. This paper was awarded the best presentation award at the ISMIR 2018 conference (the foremost international conference in MIR)
-
Best internship award from the Polytechnique school. One of the intern hired on this project funding in 2018 (Clément Tabary) received the best internship award from the French Polytechnique school for his work on unsupervised learning for musical timbre transfer.
-
Development of open-source software. The work for this research project produced different open-source software, namely Orchidea (a modular toolbox for computer-assisted orchestration) and FlowSynth (a system able to organize any synthesizer based on its timbre properties) that are both distributed freely for the general public. These softwares are used in future compositions detailed in next point.
https://forum.ircam.fr/projects/detail/orchidea/
https://github.com/acids-ircam/flow_synthesizer
-
Participation to contemporary pieces creation. Based on the researches of this project, several musical pieces are currently being created and will be performed in renowned venues. Collaborating composers include Brice Gatinet, Carmine Cella, Karim Haddad and Alexander Schubert. Some of these pieces will be scheduled to be played at the Pompidou Center and other renowned venues.
-
Special TV show on ARTE. The French TV channel ARTE programmed a show around AI and creativity which will feature a very large presence of the technologies that were developed throughout this project. The show will be broadcast on October 23rd in the Xenius series https://www.arte.tv/fr/videos/086129-015-A/xenius-les-ordinateurs-remplaceront-ils-les-artistes/
DETAILED BIOGRAPHY
Journal papers
Lembke, S.-A., Parker, K., Narmour, E. & McAdams (2019). Acoustical correlates of perceptual blend in timbre dyads and triads. Musicæ Scientiæ, 23(2), 250-274. doi:10.1177/1029864917731806
Déguernel, K., Vincent, E. & Assayag, G. (2019). Learning of hierarchical temporal structures for guided improvisation. Computer Music Journal [accepted].
McAdams, S., Goodchild, M. & Soden, K. (2019a). A taxonomy of orchestration devices related to auditory grouping principles. Music Theory Online [submitted].
Thoret, E., Caramiaux, B., Depalle, P. & McAdams, S. (2019). Neuromimetic modeling of context effects in perceived differences among complex sounds. PNAS [submitted].
Fischer, M., Soden, K., Thoret, E., Montrey, M. & McAdams, S. (2019a). The effect of orchestral timbre on the degree of perceptual segregation in real music. Music Perception [submitted].
Déguernel, K., Vincent, E. & Assayag, G. (2018). Probabilistic factor oracles for multidimensional machine improvisation. Computer Music Journal, 42(2), 52-66.
International peer-reviewed conferences
[* - invited, † - keynote address, A - peer-reviewed abstract, FP - peer-reviewed full paper]
2019
†McAdams, S. (2019). Auditory scene analysis as a tool for modeling perception of orchestration. CogMIR 2019, Brooklyn College, Brooklyn, NY.
*Esling, P. (2019). Artificial Creative Intelligence and Data Science (ACIDS) team at IRCAM. Ars Electronica Festival, Linz, Austria.
*Esling, P. (2019). Directions for the future of artificial creativity. UNESCO Conference on the future of Artifcial Intelligence, Paris, France.
*Esling, P. (2019). Variational inference and generative models for musical improvisation. Native Instruments, TU Berlin, Germany.
*Fischer, M., Soden, K., Thoret, E., Montrey, M. & McAdams, S. (2019b). The role of timbre in perceptual segregation in orchestral music. Society for Music Perception and Cognition, New York, NY. [A]
*McAdams, S. (2019). Auditory scene analysis in action in orchestration practice. Hearing (Musical) Scenes: International Symposium on Auditory Scene Analysis in Music and Speech, Hanse Wissenschaftskolleg, Institute for Advanced Study, Delmenhorst, Germany.
*McAdams, S., Goodchild, M. & Soden, K. (2019b). Orchestration analysis from the standpoint of auditory grouping principles. Society for Music Perception and Cognition, New York, NY. [A]
Antoine, A., Depalle, P. & McAdams, S. (2019). On computationally modelling the perception of orchestral effects using symbolic score information. 2019 Auditory Perception, Cognition, and Action Meeting, Montreal. [A]
Antoine, A., Depalle, P., Macnab-Séguin, P. & McAdams, S. (2019a). Identifying orchestral blend effects from symbolic score data. CogMIR 2019, Brooklyn College, Brooklyn, NY. [A]
Antoine, A., Depalle, P., Macnab-Séguin, P. & McAdams, S. (2019b) Modeling human experts' identification of orchestral blends using symbolic information. 14th International Symposium on Computer Music Multidisciplinary Research, Marseille. [FP]
Aouameur, C., Esling, P., & Hadjeres, G. (2019). Neural drum machine: An interactive system for real- time synthesis of drum sounds. International Conference on Computational Creativity, Charlotte, North Carolina, USA. [FP]
Bitton, A., Esling, P., Caillon, A., & Fouilleul, M. (2019). Assisted sound sample generation with musical conditioning in adversarial auto-encoders. International Conference on Digital Audio Effects DaFX 2019, Birmingham, UK. [FP]
Chemla–Romeu-Santos, A., Esling, P., Haus, G. & Assayag G. (2019). Multimodal signal transcription through joint latent spaces. International Conference on Digital Audio Effects DaFX 2019, Birmingham, UK. [FP]
Ducher, J.-F. & Esling P. (2019). Folded CQT RCNN for real-time recognition of instrument playing techniques, 20th International Music Information Retrieval Conference (ISMIR), Delft, NL. [FP]
Esling, P., Masuda, N. Bardet, A. Despres, R. and Chemla--Romeu-Santos A. (2019). Universal audio synthesizer control with normalizing flows. International Conference on Digital Audio Effects DaFX 2019, Birmingham, UK. [FP]
Kazazis, S., Depalle, P. & McAdams, S. (2019). A meta-analysis of timbre spaces based on the temporal centroid of the attack. 2019 Auditory Perception, Cognition, and Action Meeting, Montreal. [A]
2018
†McAdams, S. (2018). 'What is timbre?' vs. "What can we do with timbre?' Picking the right questions. Timbre 2018: Timbre Is a Many-Splendored Thing, Montreal.
*Esling, P. (2018). Artificial creative intelligence and generative variations. STIC AmSUD Symposium on Artificial Intelligence, Bucaramanga, Colombia.
Bitton, A. Chemla-Romeu-Santos, A. & Esling, P. (2018). Timbre transfer between orchestral instruments with semi-supervised learning. Timbre 2018: Timbre Is a Many-Splendored Thing, Montreal. [A]
Carsault, T., Nika, J. & Esling, P. (2018). Using musical relationships between chord labels in Automatic Chord Extraction tasks. 19th International Music Information Retrieval Conference, Paris. [FP]
Cella, C. & Esling, P. (2018). A toolbox for modular computer-assisted orchestration, Timbre 2018: Timbre Is a Many-Splendored Thing, Montreal. [A]
Crestel, L., Esling, P., Ghisi, D. & Meier, R. (2018). Generating orchestral music by conditioning SampleRNN, Timbre 2018: Timbre Is a Many-Splendored Thing, Montreal. [A]
Esling, P., Chemla--Romeu-Santos, A. & Bitton, A. (2018a). Generative timbre spaces: regularizing variational auto-encoders with perceptual metrics. International Conference on Digital Audio Effects DaFX 2018, Aveiro, Portugal. [FP]
Esling, P., Chemla--Romeu-Santos, A. & Bitton, A. (2018b). Bridging audio analysis, perception and synthesis with perceptually-regularized variational timbre spaces. 19th International Society for Music Information Retrieval Conference, Paris. [FP]
https://youtu.be/cYlryLxJZqw
Thoret, E., Caramiaux, B., Depalle, P. & McAdams, S. (2018). A computational meta-analysis of human dissimilarity ratings of musical instrument timbre. Timbre 2018: Timbre Is a Many-Splendored Thing, Montreal. [A]
Thoret, E., Caramiaux, B., Depalle, P. & McAdams, S. (2018). Human dissimilarity ratings of musical instrument timbre: A computational meta-analysis. 175th Meeting of the Acoustical Society of America, Minneapolis, MN. [A]
2017
Crestel, L. & Esling, P. (2017). Live Orchestral Piano, a system for real-time orchestral music generation. International Computer Music Conference, Shangai, China. [FP]
Crestel, L., Esling, P., Heng, L., & McAdams, S. (2017). A database linking piano and orchestral MIDI scores with application to automatic projective orchestration. 18th International Society for Music Information Retrieval, Suzhou, China. [FP]
Talbot, P., Agon, C. & Esling, P. (2017). Interactive computer-aided composition with constraints. 43rd International Computer Music Conference, Shangai, China. [FP]
Others
Macnab-Séguin P. & Lafortune, D. (2019) XML protocol for the Makimono project. MPCL Technical Report MPCL-002 (https://www.mcgill.ca/mpcl/files/mpcl/macnab-seguin_2019_mpcl-002.pdf)
McAdams, S. & Goodchild, M. (2018). Perceptual evaluation of the recording and musical performance quality of DOSim and OrchSim orchestral simulations compared to professional recordings. MPCL Technical Report MPCL-001. (https://mcgill.ca/mpcl/files/mpcl/mcadams_2018_mpcl.pdf)
Course Syllabus
Task 1: Organization and modelling of multimodal orchestral knowledge
-
Formalization of orchestral techniques from symbolic representations
The first objective is to create computational models of symbolic orchestral scores and aural analyses of the various orchestration effects categorized according to different auditory grouping processes.
-
Evaluation of signal representations for predicting orchestral effects
The second subtask aims to evaluate computational models of timbre representations, applied to multi-track audio renderings of orchestral music.
-
Perceptual assessment of formalized knowledge on orchestral effects
An extensive perceptual measurement campaign on targeted excerpts will be conducted in the third subtask to determine the relative strengths of the identified orchestration effects in simulated high-quality audio as opposed to commercial recordings
Task 2: Multimodal embedding space learning for knowledge inference
-
Joint signal-symbol embedding space and multivariate time series
The aim of this subtask is to conceive of models able to learn embedding spaces linking symbolic, acoustic, and perceptual sources of information.
-
Dimension selection through variational multimodal learning
This subtask aims to identify and discriminate information-carrying dimensions. To that end, we will define measures derived from information geometry and topology to automatically evaluate the information content of various dimensions
-
Multimodal regularities and semantic inference
In the context of orchestration, this would allow us to find audio signals with identical timbre properties but that come from widely different musical notations (different scores leading to a similar perceptual effect). This could provide a computer-assisted orchestration software that could orchestrate any given sound signal. A wide set of first-of-their-kind tasks will also be explored from this multimodal inference.