Heading section
Universal audio synthesizer control
Using deep learning to understand and control musical audio synthesizers
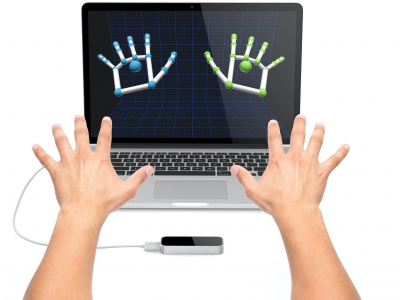
FlowSynth - Deep synthesizer control
Sound synthesizers are pervasive in music and they now even entirely define new music genres. However, their complexity and sets of parameters renders them difficult to master. We created an innovative generative probabilistic model that learns an invertible mapping between a continuous auditory latent space of a synthesizer audio capabilities and the space of its parameters. We approach this task using variational auto-encoders and normalizing flows Using this new learning model, we can learn the principal macro-controls of a synthesizer, allowing to travel across its organized manifold of sounds, performing parameter inference from audio to control the synthesizer with our voice, and even address semantic dimension learning where we find how the controls fit to given semantic concepts, all within a single model.
Project Github // Youtube video
Video demonstration
Check out our demo on YouTube
More information
Heading section
Motivations
Deep learning models have provided extremely successful methods in most application fields, by enabling unprecedented accuracy in various tasks, including audio generation. However, the consistently overlooked downside of deep models is their massive complexity and tremendous computation cost. In the context of music creation and composition, model reduction becomes eminently important to provide these systems to users in real-time settings and on dedicated lightweight embedded hardware, which are particularly pervasive in the audio generation domain. Hence, in order to design a stand alone and real time instrument, we first need to craft an extremely lightweight model in terms of computation and memory footprint. To make this task even more easier, we relied on the Nvidia Jetson Nano which is a nanocomputer containing 128-core GPUs (graphical unit processors) and 4 CPUs. The compression problem is the core of the PhD topic of Ninon Devis and a full description can be found here.
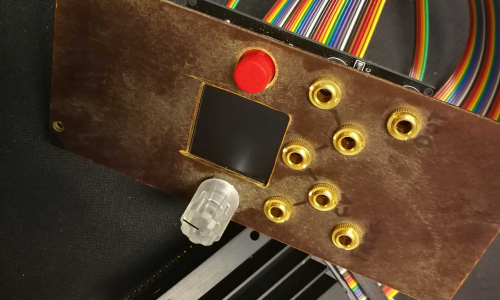
Heading section
Heading section
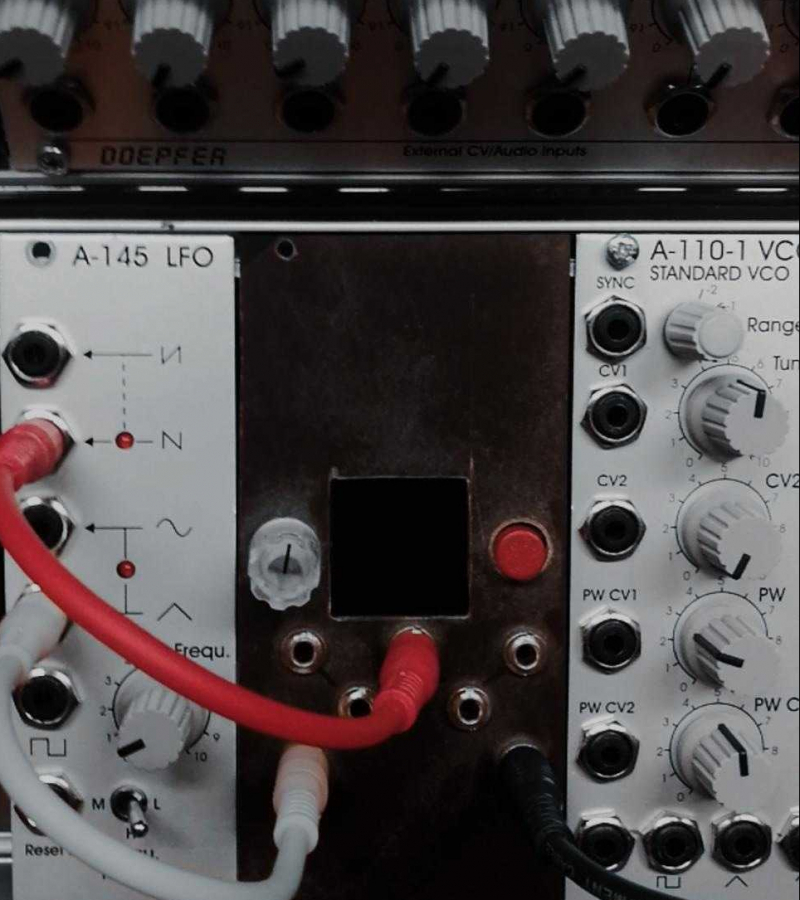
Targets of our instrument
We designed our instrument so that it follows several aspects that we found crucial:
-
Musical: the generative model we choose is particularly interesting as it produces sounds that are impossible to synthesize without using samples.
-
Controllable: the interface was relevantly chosen, being handy and easy to manipulate.
-
Real-time: the hardware behaves as traditional instrument and is as reactive.
-
Stand alone: it is playable without any computer.
Design process
The work was divided between three major steps
We set our sights on the generation of impacts as it is a very complex sound to reproduce.
Heading section
Heading section
Model Description
We set our sights on the generation of impacts as they are very complex sounds to reproduce and almost impossible to tweak. Our model allows to generate a large variety of impacts, and enables the possibility to play, craft and merge them. The sound is generated from the distribution of 7 descriptors that can be adjusted (Loudness - Percussivity - Noisiness - Tone-like - Richness - Brightness - Pitch).
Interface
One of the biggest advantage of our module is that it can interact with other synthesizer. Following the classical conventions of modular synthesizers, our instrument can be controlled using CVs (control voltages) or gates. The main gate triggers the generation of the chosen impact. Then it is possible to modify the amount of Richness and Noisiness with two of the CVs. A second impact can be chosen to be "merge" with the main impact: we will call this operation the interpolation between two impacts. Their amounts of descriptors are melt to give an hybrid impact. The "degree of merging" is controlled by the third CV, whereas the second gate triggers the interpolation.
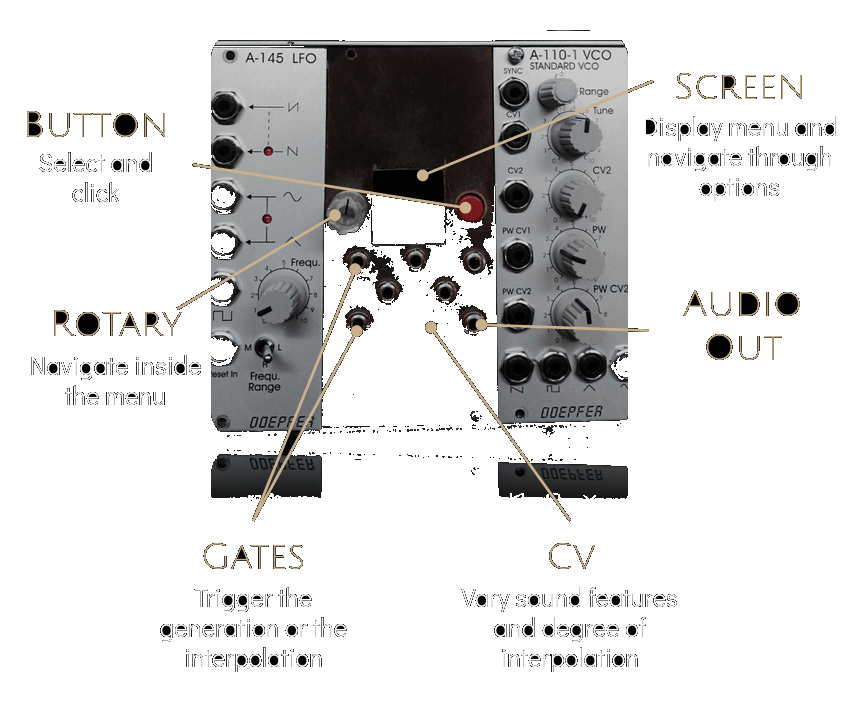
Heading section
Audio example
Here is an example of a track, where all impacts were composed with the Neurorack:
References
Esling, P., & Devis, N. (2020). Creativity in the era of artificial intelligence. arXiv preprint arXiv:2008.05959.
Esling, P., Devis, N., Bitton, A., Caillon, A., & Douwes, C. (2020). Diet deep generative audio models with structured lottery. arXiv preprint arXiv:2007.16170.